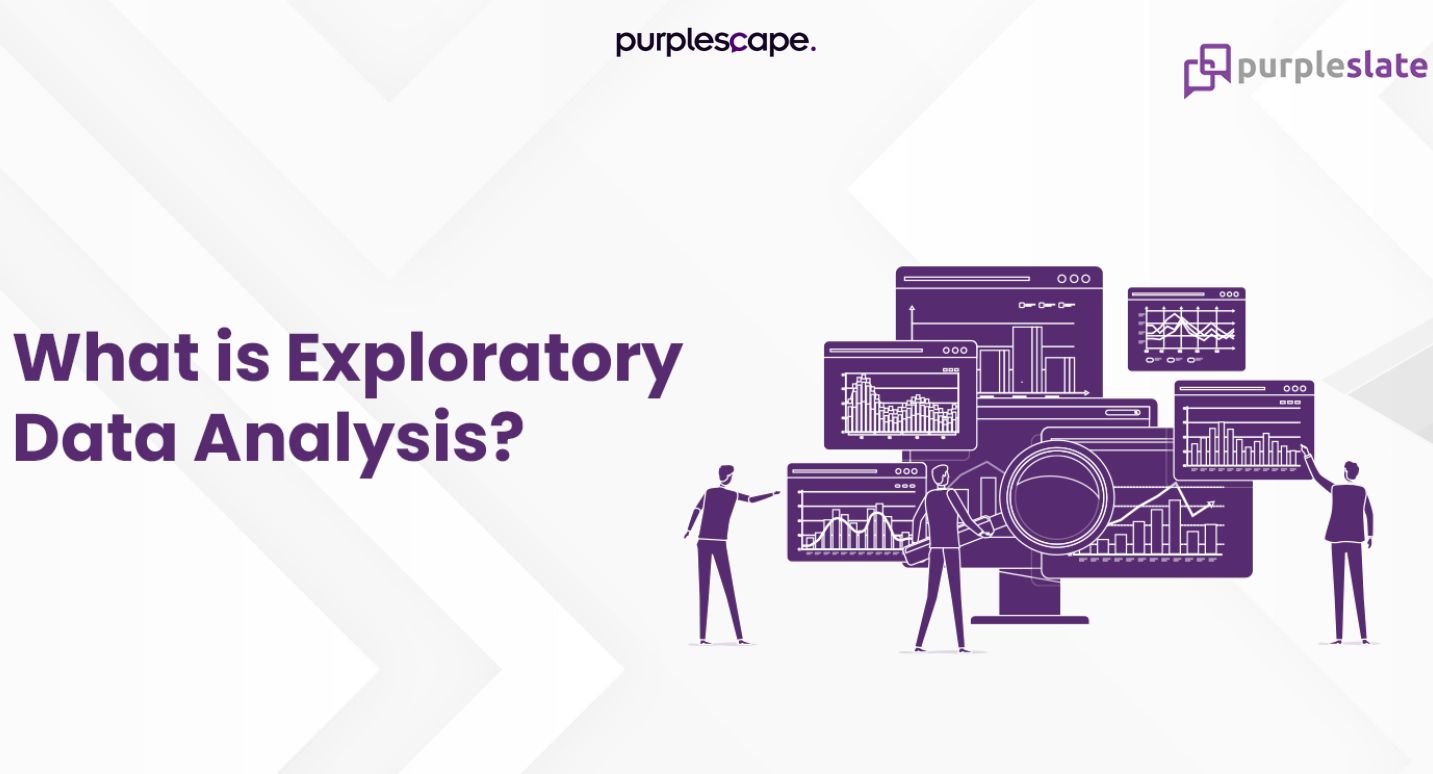
What is an Exploratory Data Analysis?
Exploratory Data Analysis (EDA) is the process of analyzing a data set without making any assumptions about its structure. In exploratory data analysis, one tries to unveil hidden patterns in the data set. The main benefit of using EDA over traditional methods like regression analysis or cross-tabulation analysis is that we can identify relationships between variables without knowing about our sample population or even existing statistical models themselves like linear regression.
In exploratory data analysis, we start with simple summary statistics that describe the dataset and then use these summary statistics to identify interesting features. We can also use these summary statistics to identify outliers and other features that are not easily identified by looking at the raw data alone.
Why do Businesses Rely on Exploratory Data Analysis?
Exploratory Data Analysis is a process that helps you to better understand your data by exploring and analyzing it. The goal of the analysis is to identify patterns in your data, find anomalies, and generate new hypotheses.
EDA is used when you want to gain more information about your dataset than can be obtained using just descriptive statistics such as mean or standard deviation. For example, You may want to estimate how many people live in a city based on census data from its population total at different ages. If there were 1 million people aged 25-29 years old living in Chicago last year (and they had an average income), then it would be reasonable to assume that there are approximately 1 million young adults aged 25–29 living somewhere else today—but how many? These questions with traditional statistical methods prove more complex, but EDA simplifies the process here.
Exploratory Data Analysis is used in many fields such as medicine, business, and engineering. It can be applied to any type of data including text-based or images. EDA helps to find hidden patterns in the data set and missing values, understand the distribution of variables, and identify correlations between variables.
How do we Perform Exploratory Data Analysis?
Exploratory data analysis is also called descriptive statistics when it involves descriptive models and less often called inductive statistics when it involves an inductive approach such as clustering algorithms. It’s simple to perform as it doesn’t require any prior knowledge about the data set at hand. The steps outlined here are common across any data practice and not just limited to EDA.
Problem Statement: The problem statement is the first step in an EDA. It consists of a brief description of the problem and its scope.
Objectives: The objectives are what the team hopes to achieve by performing the EDA, they should be SMART (specific, measurable, achievable, realistic, and time-bound).
Strategy Identification: Strategy identification is where the team decides on which strategies will be used to solve this problem.
Solution Identification: This step involves brainstorming all possible solutions that can be applied to solve this problem and then selecting one or more of them for implementation in this project.
Evaluation of Solutions: This is where each solution is evaluated against the objectives set out at the beginning of this process with a score assigned based on how well it meets those objectives; any solution that does not meet them fully should not be implemented
What are some of the common types of Exploratory Data Analysis Tools?
Exploratory data analysis can be nominally divided into four and the tools associated with EDA fall into these four categories.
Univariate Non-Graphical
Whenever the data being analyzed has only a single variable, we don’t have to dive deep and analyze causes or relationships. Such EDA methodology is called univariate non-graphical and it deals with fixed patterns that exist within the data.
Univariate Graphical
Again the variable here is single, but the representation of data patterns moves into visualization as opposed to non-graphical methods. Stem and leaf plots that describe the shape of the data distribution, histograms displaying the count (or frequency) of distribution, and box plots visualizing the median and mode are some examples of univariate graphical methods.
Multivariate Non-Graphical
If there is more than one variable at play, multivariate EDA comes into the picture. The non-graphical methodology shows the relationship between the variables through cross-tabulation or statistics.
Multivariate Graphical
The graphical representation of multivariate EDA has many ways to represent data. The most common one is the grouped bar chart with each group representing one level of the variables. Scatter plots are used to plot data points across both the horizontal and vertical axis, bubble chart visualizes data as circles based on the frequency with the larger volume being the bigger circle, and so on. We also have heatmaps that use distinguished colors to display the data values.
Final Thoughts
EDA helps everyone remove any assumptions surrounding data and is one of the foremost players in the world of data-driven decision-making. It’s a great methodology used to identify errors in data, find outliers or anomalies, and show new relationships between variables that would not have been found before.
EDA is a must have step and its insights determine the approach for more complex data modeling and machine learning. Intrigued to know more about other data analytics topics? Head over to our data glossary.